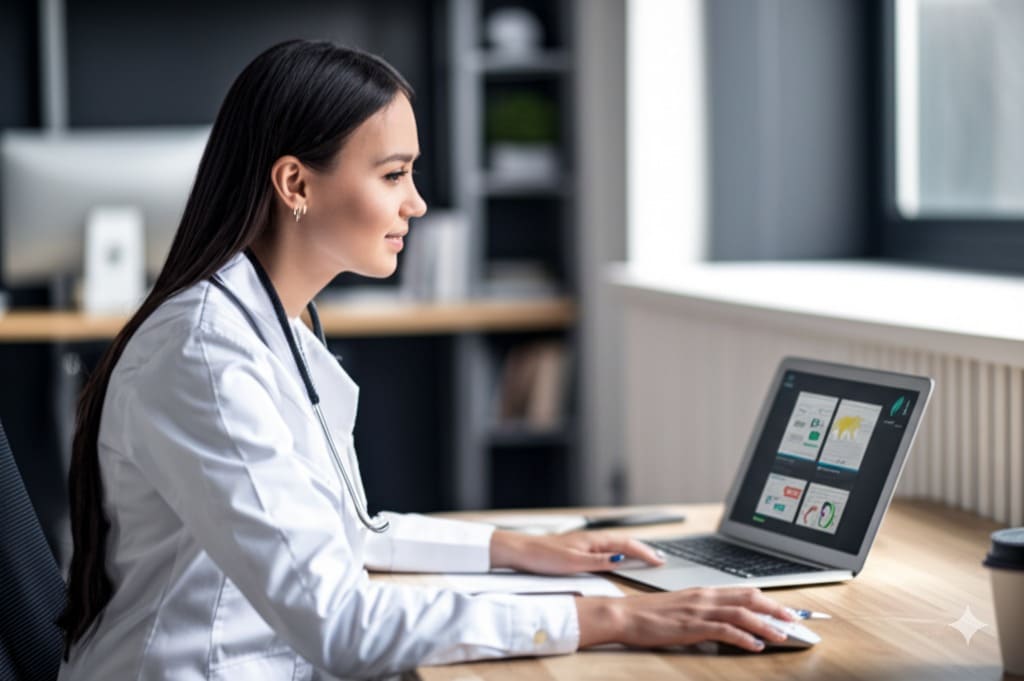
Ever felt that slight twinge of disappointment? You ask your fancy AI assistant a question, hoping for a stroke of genius, and get back… well, something kinda bland?
I’ve definitely been there.
I remember one time asking an LLM to help me brainstorm creative marketing angles for a really niche product. What I got back felt like it came straight from a generic marketing textbook, page one.
It was frustrating! I knew these tools were powerful, but I clearly wasn’t speaking their language effectively.
That little experience kicked off a journey for me, a deep dive into not just talking to LLMs, but collaborating with them.
Turns out, a few key techniques, or “patterns,” can completely transform how these models respond.
They go from simple Q&A bots to sophisticated partners that can reason, adopt expert perspectives, remember crucial details, and navigate complex decisions.
I’ve spent a good chunk of time experimenting, tweaking, and frankly, being amazed by the results.
I wanted to share four specific prompt patterns that have genuinely changed the game for me. These aren’t just theories, they are practical approaches you can start using today.
I’ll even give you some examples you can adapt.
My goal is to help you feel that “Aha!” moment I experienced when these models started working with me, not just for me.
Ready to inject some steroids into your LLM interactions? Let’s go!
Key Takeaways
Before we get into the nitty-gritty, here are the main things I hope you’ll walk away with:
- Guidance is Key: LLMs perform significantly better when you guide their thinking process, not just ask a question.
- Specific Patterns Work: Techniques like Chain of Thought, Persona, Working Memory, and Decision Trees unlock specialized capabilities.
- Iteration Makes Perfect: Getting great results is often a process of refining your prompts based on the responses you get.
1. Thinking Step-by-Step: The Chain of Thought (CoT) Pattern
Imagine you need to solve a tricky math problem. You wouldn’t just jump to the answer, right? You’d break it down, step-by-step. The Chain of Thought (CoT) pattern basically asks the LLM to do the same.
What’s the Magic Behind It?
LLMs, much like us humans, handle complex reasoning tasks better when they think methodically. Asking them to “think step by step” or “show their work” forces them to lay out their reasoning process.
This approach dramatically improves accuracy, especially for tasks involving logic, math, or multi-step instructions. Plus, it makes the model’s thinking transparent, so you can see exactly where it might have gone wrong.
Studies like the one by Wei et al. (2022) demonstrated how this significantly boosts reasoning abilities in LLMs.
Example Time!
Let’s use the probability question from my notes:
Simple Prompt:
In a group of 70 people, 40 like chocolate, 35 like vanilla, and 20 like both. How many people don’t like either flavor?
Improved CoT Prompt:
I need to solve this probability question: In a group of 70 people, 40 like chocolate, 35 like vanilla, and 20 like both. How many people don’t like either flavor?
Please solve this step by step, showing all of your work and reasoning before providing the final answer.
The difference in the quality and reliability of the response is often night and day. The LLM will typically outline:
- Identify knowns (Total, Chocolate only, Vanilla only, Both).
- Calculate the number liking at least one flavor using the principle of inclusion-exclusion:
Likes Chocolate + Likes Vanilla - Likes Both
. - Subtract that number from the total to find those who like neither.
How to Adapt CoT:
- Add phrases like
Think step by step
,Work through this systematically
, orShow all your work
. - For complex decisions, try
Consider each factor in sequence before making a recommendation
.
Here’s another quick example for a business scenario:
Improved Prompt Example (Business Decision):
<general_goal>
I need to determine the best location for our new retail store.
</general_goal>
<data>
- Location A: 2,000 sq ft, $4,000/month, 15,000 daily foot traffic
- Location B: 1,500 sq ft, $3,000/month, 12,000 daily foot traffic
- Location C: 2,500 sq ft, $5,000/month, 18,000 daily foot traffic
</data>
<instruction>
Analyze this decision **step by step**. First calculate the cost per square foot for each location. Then, calculate the approximate cost per potential customer (using daily foot traffic). Finally, consider qualitative factors like potential visibility or neighborhood compatibility (you can make reasonable assumptions if needed). Show your reasoning at each step before making a final recommendation.
</instruction>
Using tags like <data>
and <instruction>
can sometimes help structure the input, particularly for models tuned to recognize them (though results can vary).
2. Putting on the Expert Hat: The Expertise Persona Pattern
What if you need advice not just from an AI, but from a seasoned expert? The Expertise Persona pattern involves telling the LLM to adopt the mindset, knowledge, and communication style of a specific professional.
Why Does This Work So Well?
Assigning a persona helps the LLM access more domain-specific knowledge. It starts using the right terminology, applies relevant frameworks, and considers nuances specific to that field. You’re essentially focusing its vast knowledge base onto a particular area of expertise.
Example Time!
Simple Prompt:
I need help building a sentiment analysis model for customer reviews. What approach should I use?
Improved Persona Prompt:
I’d like you to respond as a senior software architect with 20+ years of experience in scalable systems and a track record of migrating legacy applications to cloud infrastructure. You take a pragmatic approach that balances technical debt reduction with business continuity.
My company has a 15-year-old Java monolith application handling our core business processes. We need to modernize it while keeping it operational.
What migration strategy would you recommend, what pitfalls should we watch for, and how would you structure the team to execute this transition? Please provide actionable advice based on your extensive experience.
Notice the specifics: years of experience, specialization (scalable systems, cloud migration), and even approach (pragmatic, balancing tech debt/business continuity).
Asking “I’d like you to respond as…” often yields a more conversational, human-like expert response compared to just starting with “Act as…”.
The kind of detailed, structured response you can get (like the example strategy involving the Strangler Fig pattern, API gateways, and common pitfalls outlined in my original notes) is far more valuable than a generic answer.
How to Adapt Persona:
- Be specific about the role (
Senior ML Engineer
,Constitutional Lawyer
,Pediatric Sleep Consultant
). - Add credentials (
with 15+ years of experience
,published in top journals
,specializing in X
). - Define their approach or style (
takes a practical, implementation-focused approach
,communicates complex ideas clearly to non-experts
).
3. Helping LLMs Remember: The Working Memory Technique
Ever have a long conversation with an LLM, only for it to forget key details you mentioned earlier? While modern LLMs have larger “context windows” (their short-term memory), they can still lose track. The Working Memory technique helps you explicitly define and reinforce critical information.
Why Bother If Context Windows Are Big?
Think of it like highlighting important points in your own notes. Explicitly telling the model “these are the key parameters for our discussion” signals that these details should be prioritized and referenced consistently.
It helps maintain focus and ensures recommendations align with constraints.
You’ll need a model or interface that maintains conversation history for this (like standard ChatGPT, Claude web interfaces, or API setups configured for memory).
Example Time!
Simple Prompt:
Let’s plan a marketing campaign. Budget is $15k, timeline is 6 weeks starting April 10, 2025. Target audience is SME founders/CEOs (25-40). Goal is 200 qualified leads. What channels should we use?
Improved Working Memory Prompt:
I’m planning a marketing campaign and need your ongoing assistance while keeping these key parameters in working memory:
CAMPAIGN PARAMETERS:
- Budget: $15,000
- Timeline: 6 weeks (Starting April 10, 2025)
- Primary Audience: SME business founders and CEOs, ages 25-40
- Goal: 200 qualified leads
Throughout our conversation, please actively reference these constraints in your recommendations. If any suggestion would exceed our budget, timeline, or doesn’t effectively target SME founders and CEOs, highlight this limitation and provide alternatives that align with our parameters.
Let’s begin with channel selection. Based on these specific constraints, what are the most cost-effective channels?
Now, as the conversation progresses, you can refer back: “Okay, based on the budget we established, how would you allocate funds between LinkedIn Ads and content marketing?” The LLM is primed to use those parameters.
How to Adapt Working Memory:
- Use clear headings or bullet points for key information.
- Explicitly state
Keep these details in mind throughout our conversation
orActively reference these constraints
. - Structure information logically (e.g., budget, timeline, audience, goals).
4. Navigating Complex Choices: The Decision Tree Pattern
Sometimes, a decision isn’t straightforward; it depends on multiple factors. The Decision Tree pattern guides the LLM through a structured, if/else-style analysis based on different criteria.
Why Use a Decision Tree Structure?
It forces the model to consider variables in a logical sequence. Instead of giving a single, potentially oversimplified answer, it explores different pathways based on specific conditions (like budget levels, technical skill, or user needs).
This is invaluable when the “best” choice depends heavily on the specific context. Research suggests structured prompting like this helps models handle complex planning and decision tasks more reliably.
Example Time!
Simple Prompt:
What blog platform should I use for my small media business? Consider budget, traffic, content type, and tech skills.
Improved Decision Tree Prompt:
I need help selecting the optimal blog platform for my small media business. Please create a detailed decision tree that thoroughly analyzes the following:
DECISION FACTORS:
- Budget Considerations:
- Tier A: Under $100/month
- Tier B: $100-$300/month
- Tier C: Over $300/month
- Traffic Volume Expectations:
- Tier A: Under 10,000 daily visitors
- Tier B: 10,000-50,000 daily visitors
- Tier C: Over 50,000 daily visitors
- Content Monetization Strategy:
- Option A: Primarily freemium content distribution
- Option B: Subscription/membership model
- Option C: Hybrid approach with multiple revenue streams
- Available Technical Resources:
- Level A: Limited technical expertise (no dedicated developers)
- Level B: Moderate technical capability (part-time technical staff)
- Level C: Substantial technical resources (dedicated development team)
For each pathway through the decision tree, please:
- Recommend 2-3 specific blog platforms most suitable for that combination of factors.
- Explain why each recommendation aligns with those particular requirements.
- Highlight critical implementation considerations or potential limitations.
- Include approximate setup timeline and learning curve expectations.
Additionally, provide a visual representation of the decision tree structure (e.g., using text-based formatting like indentation or markdown) to help visualize the selection process.
This level of detail pushes the LLM to provide nuanced recommendations tailored to specific scenarios (e.g., “IF Budget=Tier A AND Traffic=Tier A AND Monetization=Option A AND Tech=Level A, THEN consider Platform X, Y… because…”).
How to Adapt Decision Tree:
- Clearly list the decision factors.
- Define specific tiers, ranges, or options for each factor.
- Specify exactly what output you need for each branch of the tree (recommendations, reasoning, limitations).
- Consider asking for a visual layout.
Summary Table: 4 Prompt Patterns
Pattern Name | Core Idea | Why It Works | Best For |
---|---|---|---|
Chain of Thought | Ask the LLM to think step-by-step. | Improves reasoning, accuracy, and transparency. | Math, logic problems, multi-step instructions. |
Expertise Persona | Tell the LLM to adopt a specific expert role. | Accesses specialized knowledge, frameworks, and terminology. | Getting domain-specific advice, strategy, analysis. |
Working Memory | Explicitly define key info to remember. | Ensures continuity and adherence to constraints in conversations. | Long/complex discussions, project planning, scenarios. |
Decision Tree | Guide the LLM through if/else scenarios. | Provides structured analysis for multi-factor choices. | Selecting tools/strategies, nuanced recommendations. |
Final Thoughts
Mastering interaction with LLMs is about understanding how these models “think” and using techniques like these patterns to guide them effectively.
The best way to get good at this? Experiment!
Take these patterns, try the examples, but more importantly, start modifying them for your own tasks. Pay attention to how small changes in your prompts affect the quality and nature of the responses.
Prompt engineering is an iterative process. Don’t be afraid to tweak, refine, and try different approaches based on the results you’re getting.
When you start guiding the LLM’s process, you move beyond simple Q&A and unlock a powerful collaborator.
I hope these patterns help you experience that shift just like I did.
What prompt patterns have you found most effective? I’d love to hear about your experiences – share them in the comments below!
Sources
- Wei, J., Wang, X., Schuurmans, D., Bosma, M., Chi, E., Le, Q., & Zhou, D. (2022). Chain-of-Thought Prompting Elicits Reasoning in Large Language Models. arXiv preprint arXiv:2201.11903. Available at: https://arxiv.org/abs/2201.11903
- Google. (n.d.). Introduction to prompt design. Google Cloud Skills Boost. Retrieved from https://www.cloudskillsboost.google/paths/118/course_templates/539 (Illustrates general principles applicable across LLMs).
- OpenAI. (n.d.). Best practices for prompt engineering with OpenAI API. OpenAI Documentation. Retrieved from https://help.openai.com/en/articles/6654000-best-practices-for-prompt-engineering-with-openai-api (Provides practical tips relevant to structured prompting).